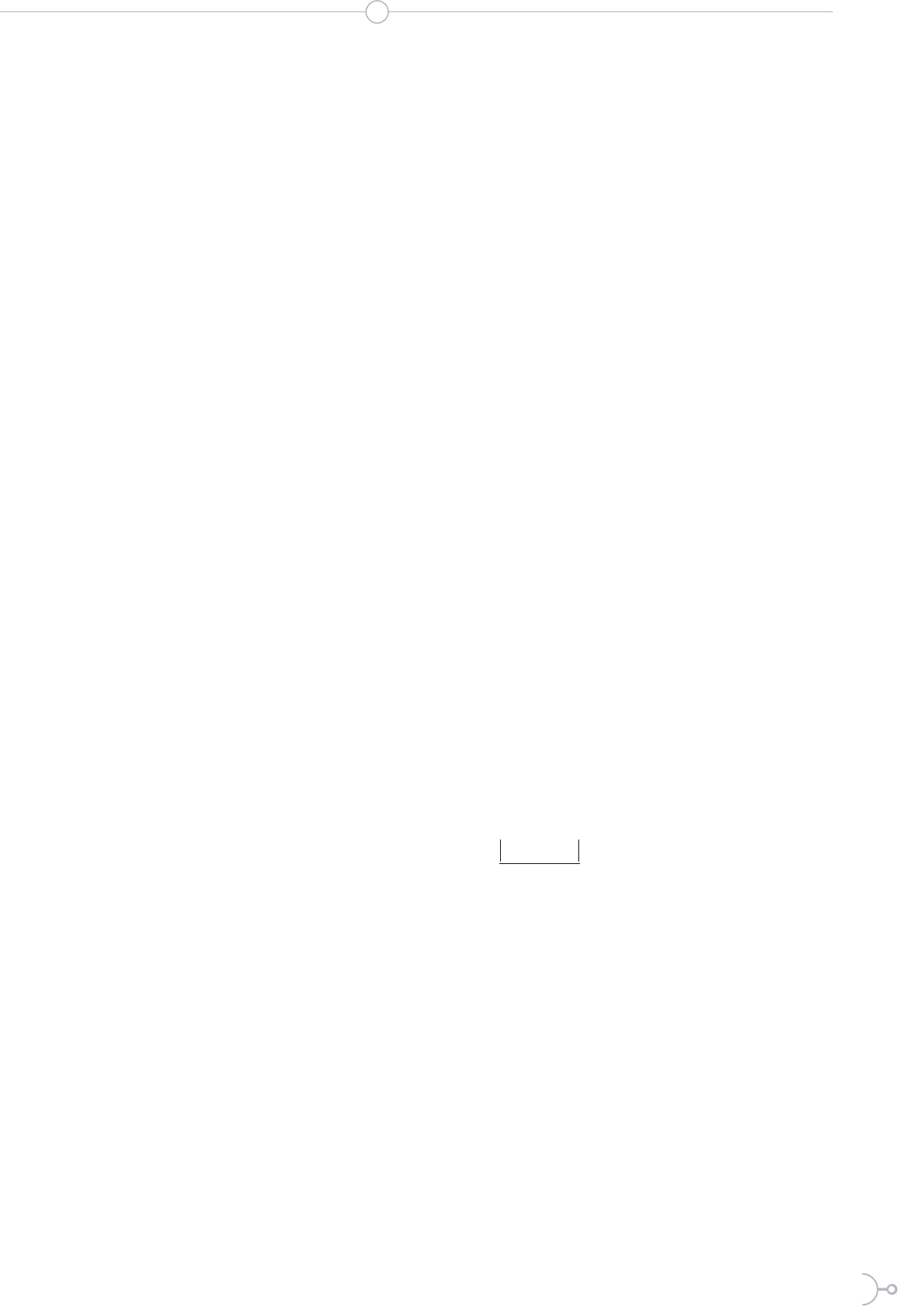
7
1. INTRODUCTION
Using solar technologies could be very
important for Ecuador since its geogra-
phical location and the presence of the
Andes Mountains makes that the solar
radiation is stronger than many other lo-
cations worldwide. In spite of their im-
portance, solar radiation measurements
in Ecuador are rare and infrequent,
mainly due to the cost of specialized
equipment and lack of trained personal.
In the existent meteorological stations,
the main problem is that the data is fre-
quently lost because the equipment is not
frequently maintained and the personal
in charge of taking the measurements is
not properly trained. For this reason, it is
important to perform statistical analysis
to have a trustworthy database (1).
Many models has been developed to
estimate solar radiation by using other
(more common) meteorological varia-
bles such as sunshine duration hours,
minimum and maximum air temperatu-
res, precipitation and wind speed (2,3,4).
Describes work global solar radiation
covering a wide range of geographical
and climate conditions; but there is not
data over Ecuador.
The main objective of this work is to de-
termine the most appropriate empirical
coefcients that are used in the afore-
mentioned models so that be possible to
impute the missing solar radiation data
in the databases.
2. METHODS
2.1 Data
Riobamba is a city situated in the cen-
tral part of Ecuador at latitude 1º 40’ 28’’
South, longitude 78º 38’ 54’’ West and
2750 m.a.s.l. An automatic meteorolo-
gical station was installed and operated
in this city between June 2007 and De-
To detect the presence of multi-variant outliers, the mini-
mum covariance determinant estimator was used. It helps
to the detection of masked outliers (1). A similar solution
was proposed by Peña and Prieto (5); in this method, the
data is projected in specific directions so that they have
high probability of showing outliers. On the other hand,
the coefficient of kurtosis is an indicator of the presence
of small groups of outliers; for this reason, the directions
where the projected points have maximum and minimum
kurtosis were calculated. Then, in these directions, all the
values in all the directions of maximum and minimum
kurtosis are tested with eq. 1. If the values surpass the
value of 5, they are considered suspects of being outliers.
Once these suspects were identified, a vector of means
cember 2012; however, the pyrometer worked until April
2012. The station was registering and recording different
parameters such as temperature, relative humidity, wind
speed, atmospheric pressure and daily average total so-
lar radiation. On the other hand, the National Meteoro-
logical Institute has installed a meteorological station in
the same city and it has provided the daily total hours of
sunshine, maximum daily temperature, minimum daily
temperature, and other parameters for the same period
of time.
The meteorological data was obtained using an automa-
tic station located at latitude 1º 39’ 17’’ South, longitude
78º 49’ 39’’ West, and 2820 m.a.s.l. The available sensors
are: pyrometer (Li-Co #LI-200SA) with certicate of ca-
libration y and error of 5%., thermometer (NGR #110S),
anemometer (NGR #40C) and pluviometer (Rain Gauge
Tipping Bucket). The average values are stored every 10
minutes in a data logger NRG Symphonie. About 950
meters from this station, it is located a manual meteoro-
logical station at latitude 1º 39’ 3’’ South, longitude 78º
41’ 7’’ West, 2840 m.a.s.l. This station has a sunshine du-
ration sensor Campbell-Stokes, which data is registered
physically.
2.2. Detection of outliers
To detect the presence of mono-variant outliers in the
data, it was performed a descriptive analysis using gra-
phics and a robust standardization using the median,
which is an estimator of the central position of the data
and the MEDA, which is a robust estimator of the disper-
sion. This calculation was performed using eq.1.
Ramos,Pérez N,Villacreses,Vaca,Chavez,Pérez M.
MEDA x
or
i
−
()
>
��